Optimizing Aerospace Data With AI
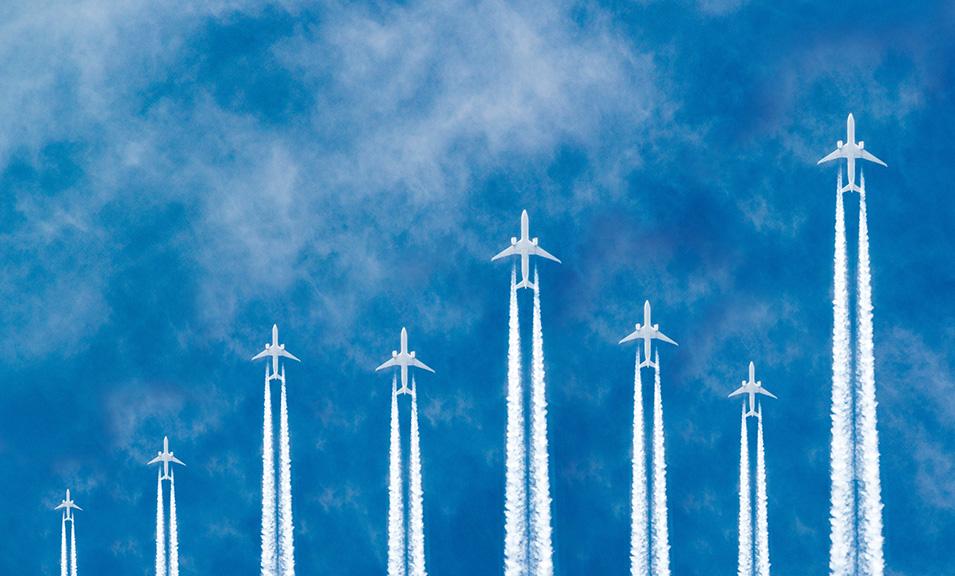
The aerospace industry has two foundational elements: accuracy and speed. The integrity of information and data and the ability to access and process this data quickly and efficiently are crucial to success within the industry, whether you are an MRO, operator, or original equipment manufacturer (OEM).
However, success is at an increased risk due to the manual data entry that is still prevalent across multiple processes and the silos between disparate systems.
Errors can occur from typos or unverified/unvalidated data.
Storing, processing, and using inaccurate data—whether a typo or using the incorrect format with another system—can significantly impact routine operations, decision-making, and the bottom line.
Leveraging AI-driven data engineering and automation solutions can improve data integrity, connect disparate processes, and mitigate the impact of inaccurate data - enabling teams to focus on strategic and value-added tasks rather than administrative tasks like validating data sets.
This whitepaper discusses how AI solutions from ePlaneAI can help aerospace companies address the challenges and impact of manual data entry, reduce data inaccuracies, and optimize data-driven processes. It will also explore how aerospace companies can modernize their infrastructure to be AI-ready and gain new levels of efficiency, compliance, and competitive advantage.
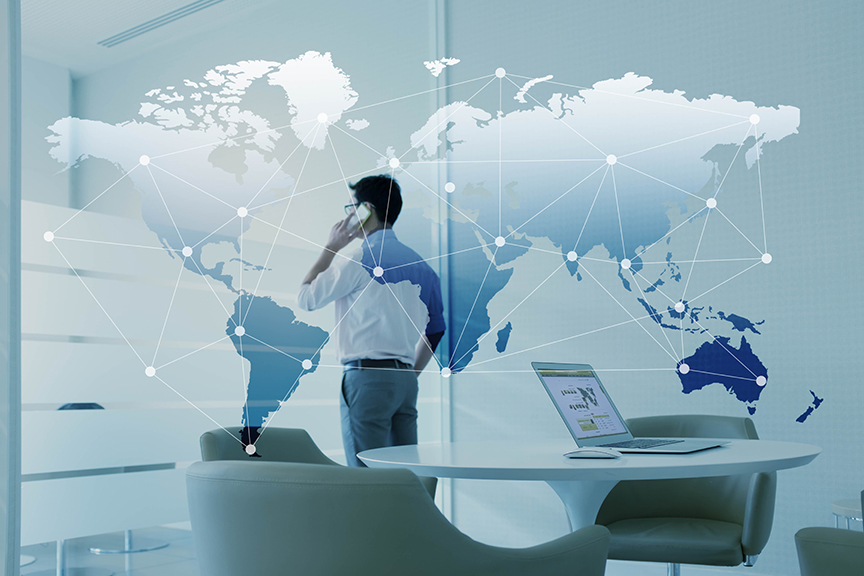
Manual Processes and The Impact of Inaccurate Data
From entering part numbers in enterprise resource planning (ERP) systems to the required information on airworthiness certificates, manual processes can inadvertently create opportunities for inaccuracies that can have significant operational and financial impacts.
Part number formatting and management are common areas impacted by manual entry errors. Due to the lack of a standard format between different systems, part numbers are often entered into databases incorrectly.
Missing information and simple typos can create duplicate part numbers across ERP systems and online marketplaces. For example, there have been up to 11 variations of a part number entered as 'AN509-C-8R4' across multiple systems such as 'AN509C-8-R-4', 'AN509C8R4', and even 'AN509-C8R-4'.
To further complicate things, each manufacturer has different part numbers, and some part numbers can have five different alternative part numbers, which makes them one line item instead of five separate line items.
When part numbers are stored incorrectly—either due to a typo or formatting error—the part becomes duplicative in the ERP or marketplace. Both types of errors prevent accurate matching, thus causing confusion for buyers and sellers when locating the correct part. A recent test conducted by ePlaneAI found that over 12.5% of unique part numbers were duplicates due to removing special characters in a one-million-part sample size.
Inaccurate part matching can hinder inventory management, specifically over-purchasing, and teams spend extensive time on administrative tasks. For example, if it takes two minutes per document to enter data into an ERP system, and there are, on average, 50 documents per day, assuming a five-day work week that equals 33 hours or 17% spent entering data, that could not be accurate with an assumed average hourly rate of $25 per hour and 50 employees working a typical five-day, 40-hour, work week, approximately $41,250 spent on potentially inaccurate data entry per month.
The lack of standardization for condition codes—a two-character abbreviation used to describe the state of aircraft components, such as whether they're factory, new, overhauled, or removed—introduces further complexity. Many mistakenly input DIST (Distributor) as a condition code instead of a customer type, which can cause inventory misclassification and ordering errors.
"There are four or five common condition codes that are used globally. We've seen at least 80 different condition codes across hundreds of thousands of aerospace parts marketplaces because there is no standard format or verification process – however the customer puts in the data is what is accepted," states Angel Marinov, Head of AI, ePlaneAI. "Instead of having a clear understanding of the condition and then ordering, there is an unnecessary step of having to validate data.”
Automating these types of data sets instead of entering the data manually helps in many ways, including reducing the amount of time spent manually inputting information, decreasing the risk of inaccuracies, and increasing the speed of autonomous data extraction/entry into systems.
Most importantly, it provides an accurate basis for the effective implementation and execution of AI. Accurate data input is the foundation of any AI tool's learning, modeling, and ultimate output. Ensuring that the tool is correct and in the correct format significantly decreases the risk of potential confusion, miscommunication, and duplicate entries.
Eliminating alternative interpretations of part numbers and codes mitigates delays, incorrect shipments, and improper ordering and helps foster a positive customer relationship.
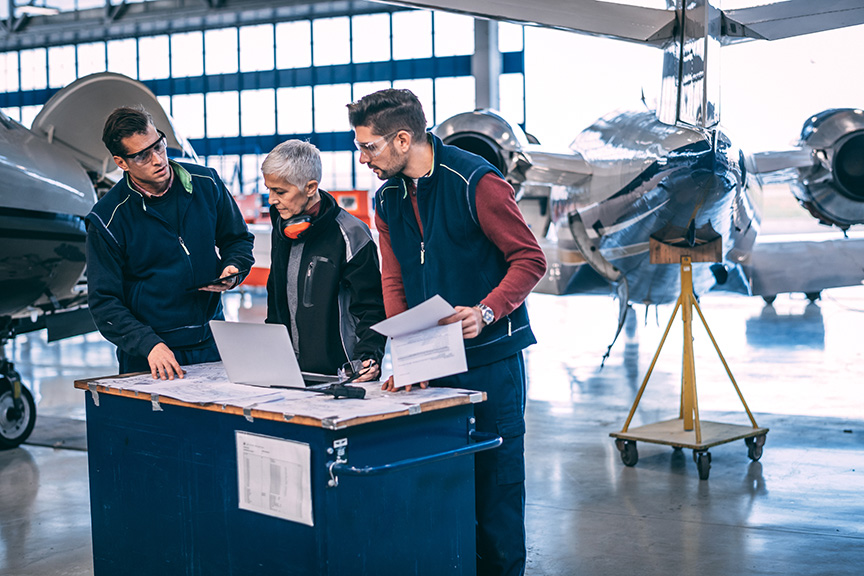
The effects of inaccurate data can cascade, impacting everything from inventory visibility to regulatory compliance.
More significantly, challenges with low-fidelity data extend to managing airworthiness certificates, which can have profound implications for compliance. Two of the 21 data fields on an airworthiness certificate are at high risk for errors: again, the part number and the date.
The part number required on an airworthiness certificate is 20 digits, exponentially increasing the possibility of entering the number incorrectly. An easy mistake such as entering an 'O' instead of a ‘0’ creates avoidable complexities.
The date field is highly risky for inaccurate data because of the lack of format standardization. One system might use a "day—month—year" format, while another might use a "year—day—month" format. Currently, very few systems can discern which format is correct. The same ePlaneAI test showed that out of one-million-part numbers, 67.31% had a date older than five years, and nearly 5% had a future date listed on the certificate.
Inaccurate certificate dates create discrepancies requiring extensive research and documentation to resolve, diverting valuable resources from core business activities. According to IDC, document automation tools can save employees approximately 2.5 hours daily on administrative tasks. For aviation, this equates to 60–80 hours saved monthly per team member, yielding $100,000 in productivity gains annually for a team of five. An Audit Costs for Compliance survey found that automating document processing can reduce audit preparation time by 50 percent. A large MRO typically spends around $50,000 annually on audit preparation; Document AI, a tool from ePlaneAI, this cost can be halved, saving $25,000 per year.
"The ERP information syncs to the digital marketplaces, so when a customer asks for this part number and the certificate information, the user goes into the ERP system to see the information," says Marinov. "If they see a date in the future or a confusing date, they need to find additional documentation to verify it. So, while it's an extra step, if the date is wrong, they cannot adjust it once the certificate has been issued. In the ERP, once you enter the data, you cannot edit it unless you’re an admin."
Inaccurate data also poses significant operational challenges and compliance risks. Discrepancies in airworthiness certificates or tracking down and verifying part numbers divert teams away from core competencies, impacting overall efficiency, competitiveness, and an organization's bottom line.
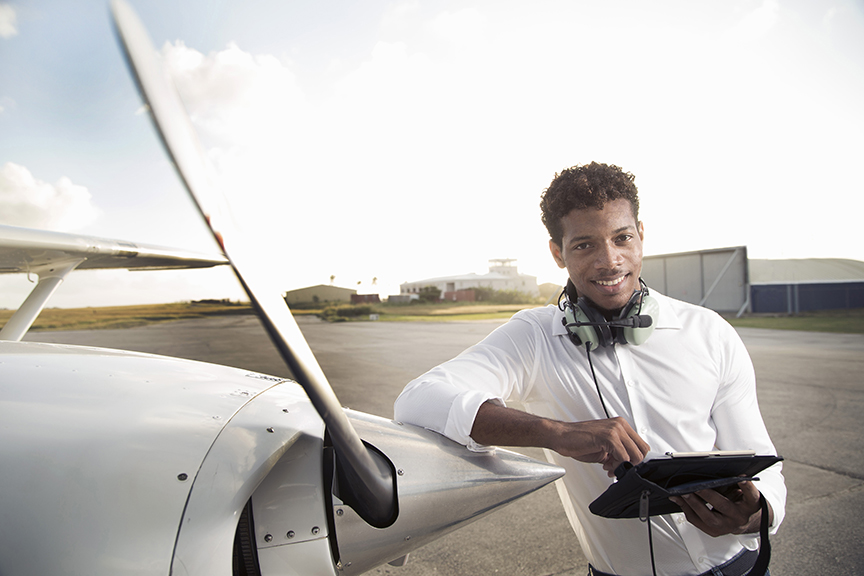
Data Engineering and Automating Data Processing to Reduce Errors
The solution to the challenges and impact of manual data entry is using NLP-driven data extraction applications to automate data entry, process, and bridge data across disparate systems. However, most processes and systems are not ready for such fast processing, nor can they connect systems that don’t “talk” to one another
ePlaneAI has developed a systematic data engineering layer that enables these legacy solutions to automate information extraction and processing in a revolutionary way, decreasing the risk of data inaccuracies.
Essentially, it is a relationship database that helps companies understand that a part number can have a subgroup of part numbers depending on the manufacturer. This database allows organizations to filter not only by part number but also by the "subgroup" to ensure all manufacturers of the same part are represented and accounted for in one central database.
Email AI and Document AI are two applications from ePlaneAI that capture, structure, and input data from various sources. These include emails, PDFs, and other unstructured formats entered into databases and ERP systems accurately and quickly. These solutions can extract data from an original source, transition the relevant information into the proper format, and enter it into an ERP system within seconds because they have been trained on hundreds of thousands/millions of emails ranging from RFQs to work order requests. Both AI applications can efficiently handle high volumes of data – over 1,000 pages simultaneously - to support large-scale aviation operations with minimal intervention. ePlaneAI has found these applications have increased accuracy by over 50 percent.
For example, Email AI streamlines email workflows, enabling users to draft, respond to, and manage emails quickly and accurately. By leveraging Large Language Models (LLMs) to read, categorize, and respond to email RFQs in 60-120 seconds, this technology can then update the ERP with both quote and party reservation details. This allows users to focus on more strategic tasks instead of their email inbox.
ePlaneAI's Document AI enables users to extract, analyze, and organize critical information from a wide range of documents, including FAA certs, IPCs, CMMs, and other essential documents. This application automates tedious manual tasks, reducing errors and significantly improving efficiency. It seamlessly integrates into an organization's existing workflow process, facilitating optimized data entry and document management that drives enhanced decision-making and operational excellence.
As with any AI application, it continually adapts to new formats and patterns, progressively enhancing its effectiveness. It becomes "smarter" by leveraging a growing repository of cross-indexed information and documents and understanding the "nuances" of each process and system.
These capabilities are possible because the data engineering layer between existing databases can transform legacy systems into AI-ready environments.
AI can understand an organization's rules, requirements, and nuances—like what data to extract and the format it needs to be in. Automating the very repeatable, day-to-day processes allows employees across the organization to be much more efficient and have the correct data for decision-making.
“We have found that more than half of databases or marketplaces contain inventory data that is over a year old, leading to wasted time, delays, and incorrect orders. With advancements in data engineering, Generative AI, and machine learning, we solve this challenge. For example, an airline using ePlaneAI’s solution alongside another database can now verify part availability in real-time. If unavailable, they can quickly find and order the correct item with a matching part number, condition code, serial number, and certificate—all within 10 days. By leveraging automation and intelligent data processing, we eliminate uncertainty and inefficiencies,” states Marinov. “The airline still needs to purchase the part, but they have the assurance that everything else is correct.
Moreover, the ability of disparate systems to "talk" to one another increases the availability of accurate information quickly, enhancing decision-making across the organization. Accurate, data-driven insights to anticipate and respond to market demands and ensure the correct parts are available to minimize disruptions and maximize customer satisfaction.
Leveraging AI solutions and an automated approach ensures that necessary data, such as part numbers, condition codes, and certificate details, are accurately recorded in a consistent format in the company's systems.
As with any industry, aerospace is highly diverse and nuanced; organizations use varying business models, operational processes, and data management practices. Through its innovative solutions, ePlaneAI has created a customizable infrastructure for real-time communication of accurate information between disparate systems.
Conclusion
The strategic use of AI and automated solutions will change how the aerospace industry processes, analyzes, and leverages data, setting a new standard for efficiency and accuracy.
Applications like Email AI and Document AI optimize aerospace data and provide precise insights for better decision-making. These tools streamline data entry and create the necessary standardization for excellent data management, providing new levels of accuracy, efficiency, and compliance for aerospace companies' most important documents.
As the aerospace industry evolves, solutions that can automate—whether AI or another form of automation—repeatable data-intensive processes will become a strategic enabler for optimizing operations and thus driving financial performance.
By embracing AI and automated technology, aerospace companies will be successful in an increasingly dynamic and data-driven landscape.
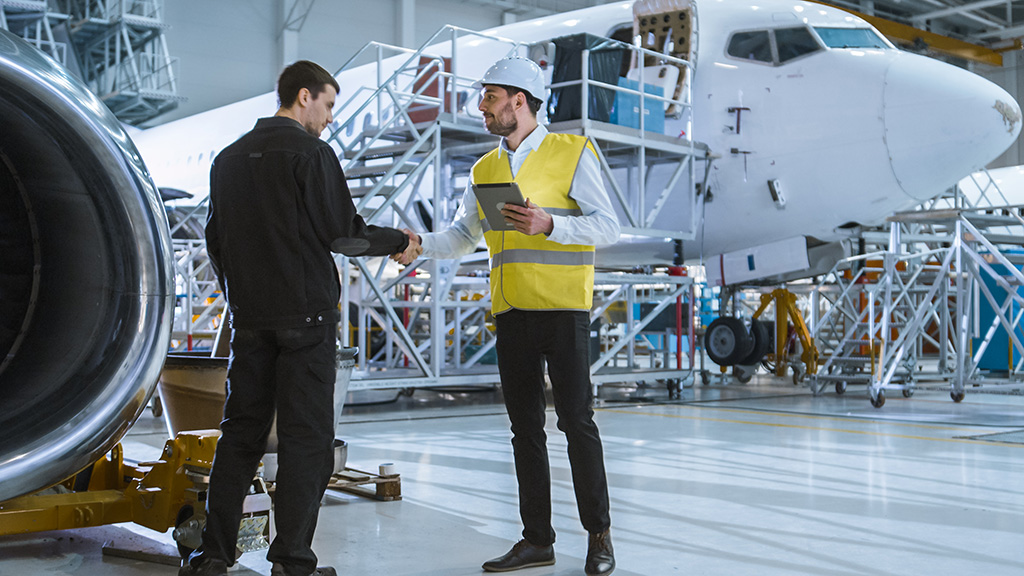