Opinion: SPAC Bonanza Drives New Challenge for Geospatial Data Analytics
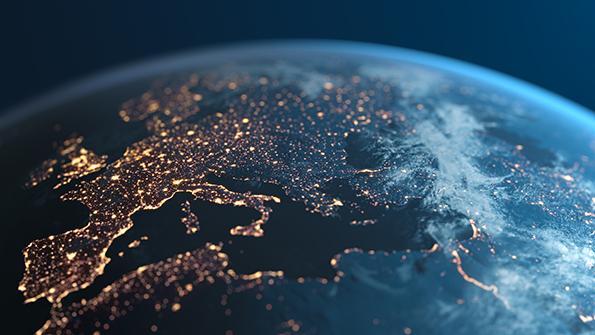
Beyond reusable launchers and microsatellites, another disruption has been happening in the space sector in the field of Earth observation. What used to be a relatively confidential, mainly institutional business has become a magnet for commercial startups and financial investors. Dozens of companies have been launched over the last decade to harness the petabytes of data coming from space and turn them into valuable information for end user communities ranging from the military to farmers and insurance brokers.
Three factors have enabled such a transformation: nanosatellite technology that has led to the proliferation of cheap space-based imaging sensors, the development of artificial intelligence (AI) and machine learning tools to automate the processing of those images, and the advent of the cloud as a new infrastructure capable of storing and computing huge quantities of data. Consequently, it is now feasible to get high-resolution images of pretty much any location or object in the world in a timely fashion, and the expectations are high for these data to be monetized into high-value information products and services.
At least that is the underlying assumption behind the recent flurry of investments involving geospatial data companies. Over the last six months alone, four such companies (Blacksky, Spire, Planet and Satellogic) have announced special purpose acquisition company (SPAC) transactions with total value of more than $5 billion, intending to raise $1.5 billion of cash in the process. This is more than seven times the amount raised annually over the last five years by all geospatial data startups combined (see chart).
While these transactions are impressive in terms of their size, they raise more questions than answers for the recipient companies and the sector as whole. It is one thing to raise money; it is another to use it wisely and profitably.
The first challenge for these companies is to decide where to play in the value chain. Should we invest in the acquisition of data through a proprietary satellite constellation? Should we focus on developing an AI-driven data processing and management platform for multipurpose analytics? Or should we integrate AI and human expertise into intelligence services for targeted end user communities?
Choosing a spot in the chain is a challenge because it is unclear yet where the value creation potential is highest. Some players are opting for a vertical integration strategy—being present on several stages of the value chain, which allows them to hedge their bets. The problem with that approach is that it is extremely difficult to manage for small companies because it typically implies a lot of fixed costs to be amortized over a still rather slim revenue base. Today, most of the money is still in the sale of raw or preprocessed data, hence the rationale to be in data generation (and ownership). But tomorrow, there will be so many data sources that this part is likely to become commoditized and therefore much less valuable.
Most players are currently focusing on developing processing algorithms to automatically generate “analytics-ready data” using AI and computer vision. Here the issue is twofold: The technology is still immature, and it could take years before something reliable enough emerges. Right now, companies are still spending a lot of resources getting the inputs right by structuring, cleaning and labeling the data before passing it through the machine learning process. Indeed, any deficiency in input quality will negate the potential benefits of AI and computer vision.
More importantly, this approach of building a large-scale generic platform (sometimes called “a data refinery”) will only be viable if an ecosystem of value-added apps and intelligence products takes shape further downstream and can be “plugged and played” on such a platform. In other words, the holy grail of a completely automated Earth data processing platform is only worth pursuing if it helps create tangible value for end users by helping them make more money or better decisions. And it is not only about scale, speed and automation. It is also about timeliness, relevance, reliability and accessibility. In one word, it is about actionability.
Thus, building an AI-driven, fully automated data processing platform is not an end in itself. It is just a means to the end of delivering a service that customers are ready to pay for. It is like in the restaurant business: You may have the best kitchen in the world, but if you don’t have a good chef and an appealing menu, you are unlikely to attract customers willing to pay a premium.