Physics-Based AI Promises To Accelerate Aerospace Design Optimization
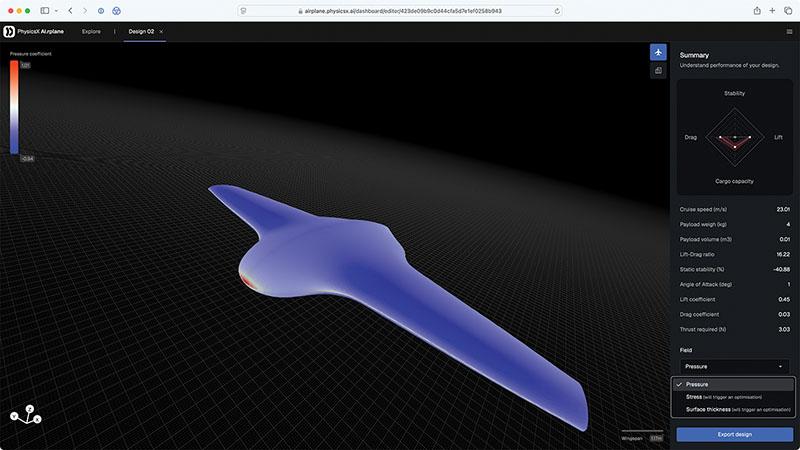
PhysicsX’s LGM-Aero AI-based analysis tool predicts performance of new shapes.
Credit: PhysicsX
Numerical simulation has become an essential part of aircraft design, from aerodynamics and structures to aeroacoustics and thermal analysis. But the escalating use of modeling and simulation comes with a cost—the tens of millions of computational core hours required to develop an aircraft...
Physics-Based AI Promises To Accelerate Aerospace Design Optimization is available to both Aviation Week & Space Technology and AWIN subscribers.
Subscribe now to read this content, plus receive critical analysis into emerging trends, technological advancements, operational best practices and continuous updates to policy, requirements and budgets.
Already a subscriber to AW&ST or AWIN? Log in with your existing email and password.